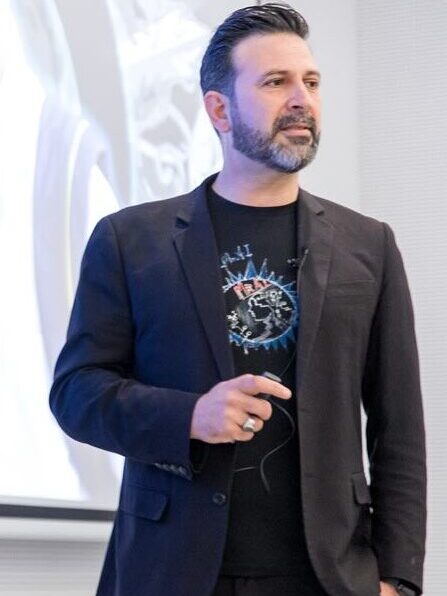
Greetings, and welcome to my personal website! I’m the Vice-Rector for Research and Innovation at Neapolis University Pafos in Cyprus, a Professor of AI, and the director of the Intelligent Systems Laboratory (isLab).
My academic background includes a Diploma (M.Eng.) in Electrical and Computer Engineering and a Ph.D. in Computer Vision, both earned from Democritus University in Greece. After completing a 6-year postdoctoral fellowship at the Center for Research and Technology Hellas (CERTH), I joined the Cyprus University of Technology as a visiting teaching staff. I also served as a visiting lecturer and researcher at the Alpen-Adria-Universität Klagenfurt in Austria. In 2017, I was elected Associate Professor at the Neapolis University Pafos.
My research focuses on the exciting intersection of Artificial Intelligence, Computer Vision, and Robotics. Over the years, I have been privileged to be involved in several R&D projects funded by European and National organizations. For my contributions, I have received several distinctions, grants, scholarships, and awards.
I am thrilled to have been involved in several innovative projects that have made significant contributions to the field, including:
- Participating in the European project sFly, we developed a pioneering visual-SLAM-based autonomous navigation mechanism for UAVs to navigate in GPS-denied environments.
- Serving as the Technical Scientific Manager for the European FP7 project NOPTILUS, where we proposed a novel methodology for the cooperative, fully autonomous navigation of teams of AUVs, which has the potential to revolutionize underwater exploration.
- Making a valuable contribution to the field of image retrieval through the development of Compact Composite Descriptors (CCDs), a set of low-level features that accurately describe and retrieve visual multimedia content.
I am also delighted to share that my research on educational robotics has been recognized for excellence by the Cyprus Education Leaders Awards in 2020, 2021, and 2023. This recognition is a testament to my unwavering dedication to advancing the field of educational robotics, and it is truly an honor to be part of such a distinguished community of educators and researchers. In addition, my research lab has achieved notable success in international educational robotics competitions, receiving numerous awards and distinctions.
I’ve been a reviewer for leading scientific journals in artificial intelligence, image processing, and multimedia (e.g., IEEE PAMI, IEEE TMM, IEEE TRO, etc.). I’m also an editorial board member in several international academic journals. Moreover, I have served as a reviewer and member of the program committees of several international conferences/workshops (e.g., IEEE IROS, IEEE ICRA, etc.). I’m also a Senior member of the IEEE and a proud Sigma Xi member.
As a software developer, I have worked on several popular, open-source, and commercial software tools for different fields, including multimedia, information retrieval, information management, and gaming. I’m one of the contributors to the LIRe library, the img(Rummager) platform, and the core developer of the Houdini MC project.
In my free time, I write children’s stories.
Current Projects
The Lagrangian remainder of Taylor’s series distinguishes O(f(x)) time complexities to polynomials or not.
This work investigates the time complexity consequences of the truncated Taylor series, known as Taylor Polynomials. In particular, it is demonstrated that examining P=NP equality is associated with determining whether the n^th derivative of a particular solution is bounded. Accordingly, in some cases, this is not true, and hence in general.
A General Framework of High-Performance Machine Learning Algorithms: Application in Structural Mechanic
This research proposes high-performance machine learning algorithms for modeling structural mechanics problems. These algorithms are implemented in parallel and distributed computing environments to handle highly demanding problems. Four machine learning algorithms are proposed and tested in four structural engineering problems. The models accurately predict target variables and effectively assess structural performance, as indicated by reduced residual errors. The proposed algorithms demonstrate the generic characteristic of the machine learning framework for structural mechanics problems.